A recent Kaggle competition winner was disqualified for stealth-cheating i.e. the results from his Machine-Learning (ML) code was actually giving the expected output using pre-determined methods, and not ML. Kaggle, a google-owned platform, is the ‘in’ platform for ML open competitions offering points, badges and significant sponsored cash prizes to the winners (a sum total of $25K in this case).
Competitions aren’t the only place where disillusionment in ML happens.
It is easy to debunk such claims by asking the right questions about the data and results. For those who are data-inclined should also look at the RoC Curve and Confusion Matrix to get more insights on the numbers published.
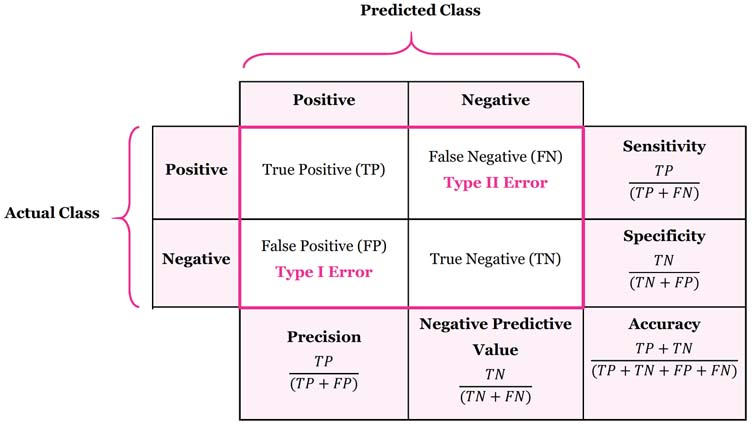
Overtly optimistic accuracy levels may also sometimes be attributed to Target Leakage (also known as Data Leakage, or sometimes Model Leakage). This simply means that we are predicting an outcome which has already happened in your training data, and that too without time travel :-). A simple example to understand this: if we are trying to predict whether a customer will make his next subscription payment on the basis on whether the customer account has been deactivated. We will, of course, get a fantastic accuracy because if the customer’s account has already been deactivated, the obvious hypothesis is that he will not make his next subscription payment. Even though this is a hugely simplistic example, but still data engineers may miss out on parameters which make the model leaky and optimistic.
Despite these disillusionments, I am big fan of futurism and believe that the future is here now! Maybe that’s the reason I easily fall prey to inflated expectations. Yes, AI and ML still have a huge potential to live up to. It’s there, lets just step ahead surely and firmly!